Case study: ExoMatter
Using AutoML in materials science to predict material properties.
17 Dec 2023 · 4 minute read
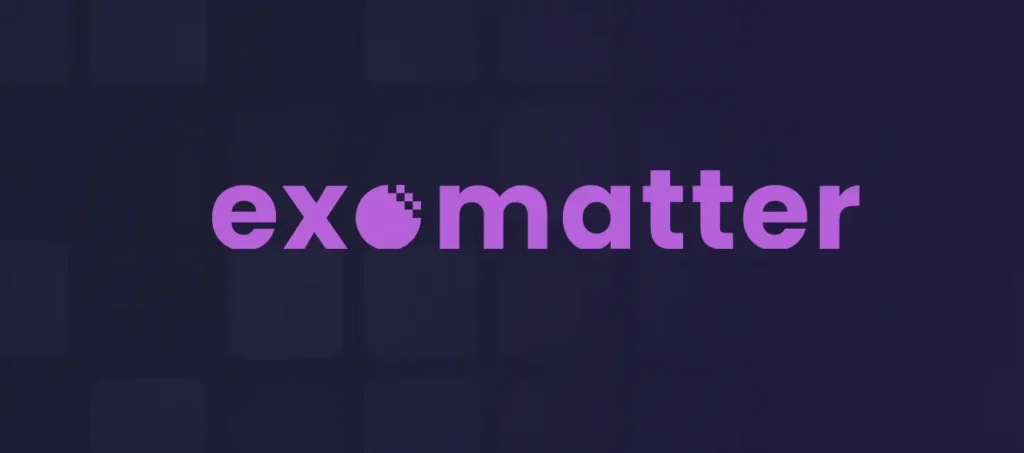
This is a case study with Josua, the CEO of ExoMatter, a company that deals with a lot of data in the material science world. We have helped them build a Automated Machine Learning MVP using Google’s Vertex AI, to make better use of their data.
Can you tell me a bit about ExoMatter?
ExoMatter is a company that deals with materials development and computation, we help companies find the right material for the specific applications, so we replace a lot of trial and error in the lab with data. That’s something we do across different industries, so it’s not limited to one specific tiype of material, and we can calculate new materials from scratch that didn’t exist yet. AI has a key role in growing the business. One thing is doing simulations and using the data that is there, but those simulations are computationally expensive, so it makes sense to use ML to predict some properties. Also some of those properties are not accessible in any other way, so we use ML to fill in the gaps. What we have been doing so far is that we’ve done some experiments building our own ML models; we have either used models that already exist, but we’ve also built our own models, and it took quite some time, to build them, structuring the data, and finding out what algorithm to use and what features are important. That’s why we thought AutoML could have been a good solution.
How did we start working and what was the process for choosing a vendor for the ML MVP?
We knew each other before, we knew what your capabilities are. I had the feeling that you could get things done quickly. We had to get quotations from other agencies for this project, but in the end yours was the most convincing. You already had some ideas on what do to, so that was quite an easy decision.
How would you describe the collaboration in a remote setting?
I would say it was quite efficient. We first had to get a standing on the project matter, as chemistry is not something someone deals with everyday, so we had to spend some time in the beginning, and then we did regular calls when something was ready. I think it was quite easy to collaborate, especially also given that I didn’t have too much time. I trusted you to give a good result, and that’s what happened. It was good.
What is your feedback on our communication?
I think it was great. Also, the documentation you did was good, so not really much to improve on that.
In the first few weeks we got the MVP working. What do you think about the tradeoff between cost, speed and quality of AutoML models?
That’s great, is was exactly what we were hoping to see. It was a pilot project, and the goal was to see if it would work or not. It could have been that it didn’t work with the data we were using. The models we built before took 3/4 months to build (for one model). Now we have this AutoML pipeline where we don’t have to do much, it trains overnight, that we tested on 2 datasets, and the results were at least as good as previous results. I’m quite happy with those results.
In the first few weeks we got the MVP working. What do you think about the tradeoff between cost, speed and quality of AutoML models?
I could not have wished for anything better, because it was really within the budget that we originally planned. In my head I always had some buffer, because we had more budget than we contracted, just to make sure in case something goes wrong we can still do it, but we did not need that kind of buffer. So in the end we used it to explore some more options to improve the data quality, which we didn’t plan originally. I’m quite happy with that.
Would you recommend our services for other similar companies and what would be the reason?
Yes, definitely. I would recommend you if someone needs short term support in AI projects. We’ve not gone beyond this AI use case, but I’m sure you can handle other things.